Traditionally, banks have been pretty cautious about jumping on the tech bandwagon. This is because integrating new technologies can be a complex process, especially when dealing with money and security. Banks today, however, are constantly evolving, and Artificial Intelligence (AI) is becoming a game-changer in the banking sector.
Why? From enhancing customer experiences to improving operational efficiency and even managing risk, AI is reshaping how the banking industry works. In fact, according to Gartner, 42% of banking CIOs have deployed or are planning to deploy AI within the next year.
This shows that AI isn't just becoming essential for improving efficiency, but is becoming a core part of how banks operate. In this blog, we’ll break down how AI is reshaping the banking industry and provide real-world use cases that highlight how powerful it can be.
Let’s dive in 👇
Why AI matter to banks?
AI is transformative for banks because it speeds up the digitisation of end-to-end processes, from customer interactions to back-office operations. With tools like data analytics and Machine Learning (ML) algorithms, AI enhances the precision and efficiency of services, and ultimately leads to improved customer satisfaction and operational excellence.
It also plays a crucial role in fraud detection and prevention. AI helps banks identify and mitigate risks before they become bigger problems. Moreover, AI-driven tools like chatbots, voice recognition and facial recognition apps are revolutionising the customer experience, making banking more accessible and secure.
As a result, banks that integrate AI are better positioned to stay competitive and meet the evolving needs of their customers in a rapidly changing financial landscape.
Use cases of AI in banking
There are many ways banks are using AI in their everyday operations:
Use case 1 - Fraud detection
Traditional methods often rely on rule-based systems that can be bypassed by criminals. AI, on the other hand, uses Machine Learning algorithms to analyse vast amounts of data in real-time and detect anything unusual that might indicate fraudulent behaviour. This allows banks to flag suspicious transactions before they can cause significant damage.
Moreover, AI systems can continuously learn and adapt to new fraud tactics over time. For instance, neural networks can be trained to detect unusual spending patterns that deviate from a customer's typical behaviour. This not only improves security but also reduces false positives.
Use case 2 - Chatbots for customer service
AI-powered chatbots are transforming customer service in the banking industry. From simple account balance checks to more complex issues like resolving payment disputes, they can handle a wide range of inquiries. And they do it in a way that feels natural and human-like by using Natural Language Processing (NLP).
Plus, chatbots can gather valuable data on customer preferences and behaviours, which banks can use to tailor their services and products more effectively. This data-driven approach enhances customer loyalty and drives business growth.
Use case 3 - Loan processing
AI is making loan processing and credit scoring faster by automating them. Traditional methods often involve manual reviews and lengthy approval processes, which can be time-consuming and prone to errors. But AI algorithms can analyse credit history, income and spending patterns in seconds to make more accurate and consistent credit decisions. This not only speeds up the loan approval process but also reduces the risk of default.
AI can also identify potential risks that might be overlooked by human reviewers. For example, Machine Learning models can detect subtle patterns in financial data that indicate a higher likelihood of default. This enhanced risk assessment helps banks to make better lending decisions while reducing exposure to bad loans.
Use case 4 - Algorithmic trading
Algorithmic trading, powered by AI, allows banks to execute trades at optimal times and prices. AI algorithms can analyse market data in real-time and identify trends that human traders might overlook. This means banks can make more informed and profitable trading decisions, leading to bigger returns and smaller risks.
AI can also automate the trading process, reducing the need for manual intervention and minimising the potential for human error. High-frequency trading (HFT) algorithms, for example, can execute trades within milliseconds, seizing opportunities that humans can’t keep up with.
Use case 5 - Personalised financial advice
AI is allowing banks to offer personalised financial advice to their customers, enhancing the overall banking experience. By analysing individual financial data, AI algorithms can provide tailored recommendations on savings, investments and budgeting. This personalised approach helps customers make more informed financial decisions that fit their financial situation.
Moreover, AI can continuously monitor a customer's financial situation and provide real-time advice on investment decisions and mortgages based on changing circumstances. For instance, if a customer's spending habits indicate a potential risk of overspending, the AI system can send them timely alerts and suggestions to help them stay on track.
Use case 6 - Portfolio optimisation
AI can optimise portfolios by providing sophisticated tools to manage investment. By analysing historical market data and current economic conditions, AI algorithms can suggest investment strategies and asset allocations. This data-driven approach helps banks maximise returns while minimising risks.
What’s even better is that AI can continuously monitor and adjust portfolios in real-time, responding to market changes and economic indicators. For example, if the market shows signs of volatility, AI can automatically rebalance the portfolio to reduce exposure to high-risk assets. This level of automation provides clients with peace of mind.
Real-world examples of AI success in banking
Several banks have already begun to leverage generative AI to achieve remarkable results. One notable example is JPMorgan Chase, which has implemented generative AI to automate the creation of legal documents and contracts. The system, known as COIN (Contract Intelligence), can review and interpret legal documents in seconds, a task that'd typically take hundreds of hours for human lawyers. It not only speeds up the document review process but also reduces the risk of errors and inconsistencies.
Another success story comes from HSBC, where its AI-powered chatbot, Amy, can generate natural and contextually appropriate responses to customer inquiries. Amy has significantly reduced the time it takes to resolve customer issues and has improved customer satisfaction scores. It can also generate personalised financial advice and recommendations, helping customers make more informed decisions about their finances.
In investment banking, Goldman Sachs has leveraged generative AI to develop sophisticated trading algorithms. These algorithms can generate and execute trading strategies based on real-time market data and historical trends. Goldman Sachs has been able to identify new trading opportunities and optimise its investment portfolios, leading to higher returns and reduced risks.
Adopting AI in banking
Now that you know the use cases of AI in banking, let’s look at some of the challenges that come with adopting it and more importantly, how banks can overcome them.
Cybersecurity risks
The increased use of AI can expose banks to new cybersecurity vulnerabilities, such as data breaches and unauthorised access to sensitive information.
To address these risks, banks must implement robust cybersecurity measures that are specifically designed to protect AI systems. Here’s how:
- Advanced encryption and secure storage - encrypting data both during transit and at rest, ensures that sensitive information stays protected, even if it’s intercepted.
- Multi-factor authentication (MFA) - adding layers of authentication makes it much harder for unauthorised users to get into AI systems.
- Proactive cybersecurity measures - regular audits, AI-driven threat detection systems and updating protocols can help banks stay ahead of hackers.
- Employee training - banks should educate their employees on identifying phishing attempts, setting strong passwords and safely handling data.
Successful implementation of AI
Successfully implementing AI in banking is a challenge as it requires a well-thought-out strategy that addresses both technical and organisational challenges. There are many ways banks can resolve this:
1 - Start small
Instead of diving headfirst into massive AI projects, banks should begin with smaller, manageable initiatives. For example, launching an AI-powered chatbot for customer service or using AI for fraud detection can deliver quick wins. These projects not only provide immediate benefits but also help banks build confidence in the technology.
2 - Test, learn and scale
Small projects allow banks to work out the kinks before expanding AI adoption. This iterative approach ensures that any technical or operational issues are resolved early.
3 - Clear objectives
Every AI project should have a clear purpose and measurable outcomes. Whether it’s reducing fraud or improving customer satisfaction, defining success metrics keeps the team focused and aligned.
Collaboration with tech firms
Collaboration between the tech and finance sectors, often enabled by fintech companies, is essential for overcoming barriers to AI adoption in banking. This is because many banks may lack the in-house talent needed to develop and implement AI systems.
By partnering with fintech companies, banks can gain access to cutting-edge AI solutions and expertise that they might not have in-house. For example, banks can collaborate with tech firms to develop custom AI solutions that address specific business needs. This might involve working with AI specialists to create advanced fraud detection systems or personalised financial advice platforms.
By leveraging the expertise of tech companies, banks can leapfrog the challenges of AI adoption and build internal capabilities to manage and scale AI initiatives.
The future of AI in banking
When it comes to the future of AI in banking, the possibilities are endless. AI isn’t just reshaping how banks operate today — it’s laying the foundation for a smarter, more efficient and customer-focused industry.
From delivering hyper-personalised customer experiences to catching fraud before it even happens, AI will touch every corner of banking operations. It’s also set to make regulatory compliance smoother and back-end processes lightning-fast.
But here’s the thing: success with AI isn’t just about having the latest tech. It’s about how well banks can integrate it into their existing systems, keep the data feeding it accurate and up to date and ensure they’re transparent about how it’s being used — and how it’s benefiting customers.
Fast forward to 2025 and beyond, and the banks that thrive will be the ones that find the perfect balance. They’ll leverage AI to do the heavy lifting while maintaining the human touch that keeps customers feeling valued and heard. The future of banking isn’t just about algorithms; it’s about using those algorithms to build trust, improve lives and keep the human connection alive.
FAQs
How does AI improve customer service in banking?
AI enhances customer service by providing 24/7 support through chatbots and virtual assistants, which handle queries like balance inquiries, transaction tracking and payment disputes.
It also personalises customer experiences by analysing preferences and offering tailored financial advice or product recommendations.
What are the main applications of AI in financial services?
Key applications of AI in financial services include fraud detection, customer service automation, loan processing, algorithmic trading, personalised financial advice and regulatory compliance. These solutions help improve efficiency, security and customer satisfaction.
How is generative AI being used in banking?
Generative AI is being used to create personalised marketing campaigns, generate customer insights and draft communication like financial reports or customer emails. It’s also aiding in scenario analysis and predictive modelling for better decision-making.
What challenges does the banking industry face with AI adoption?
Banks face challenges like integrating AI into legacy systems, ensuring data accuracy, addressing cybersecurity vulnerabilities and maintaining transparency with customers about AI usage. Additionally, regulatory and ethical concerns must be navigated carefully.
How can AI impact risk management in banking?
AI strengthens risk management by analysing vast datasets to identify potential risks, detect anomalies and predict market trends. It can also automate compliance checks, reduce human errors and provide real-time monitoring to help banks respond proactively to emerging risks.
Create robust custom software today
100s of businesses trust us to help them scale.
Book a demoBy proceeding you agree to Builder.ai’s privacy policy
and terms and conditions
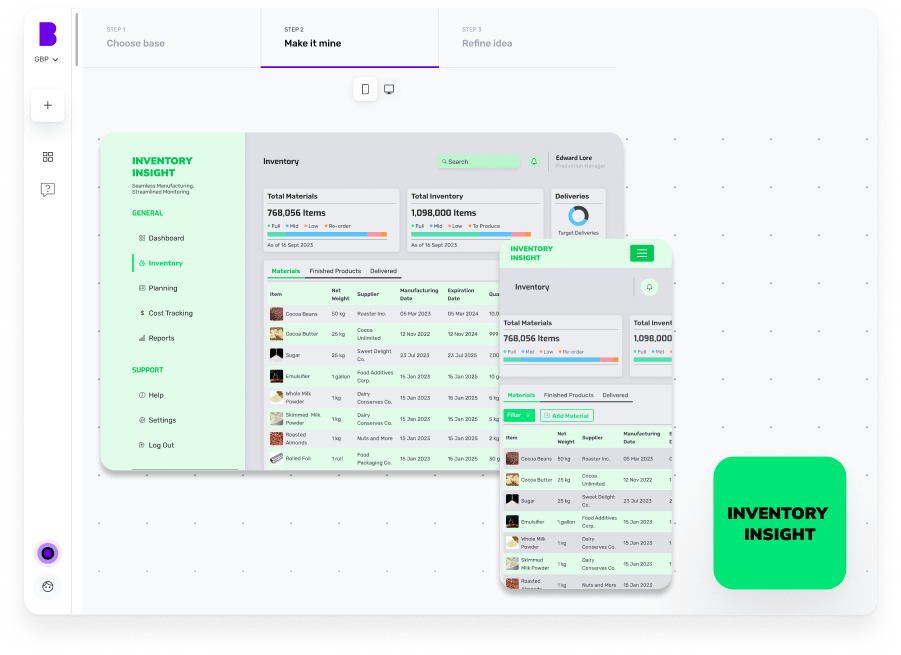
Gaurav is the SEO Content Writer at Builder.ai. Being an Engineer and Marketing MBA, he has a knack for converting technical jargon into marketing content. He has 8+ years of experience creating content and designing marketing campaigns that drive organic growth for B2B companies and tech startups.